时间:2024年5月13日13:00-14:30
地点:ZJUI大楼 B407
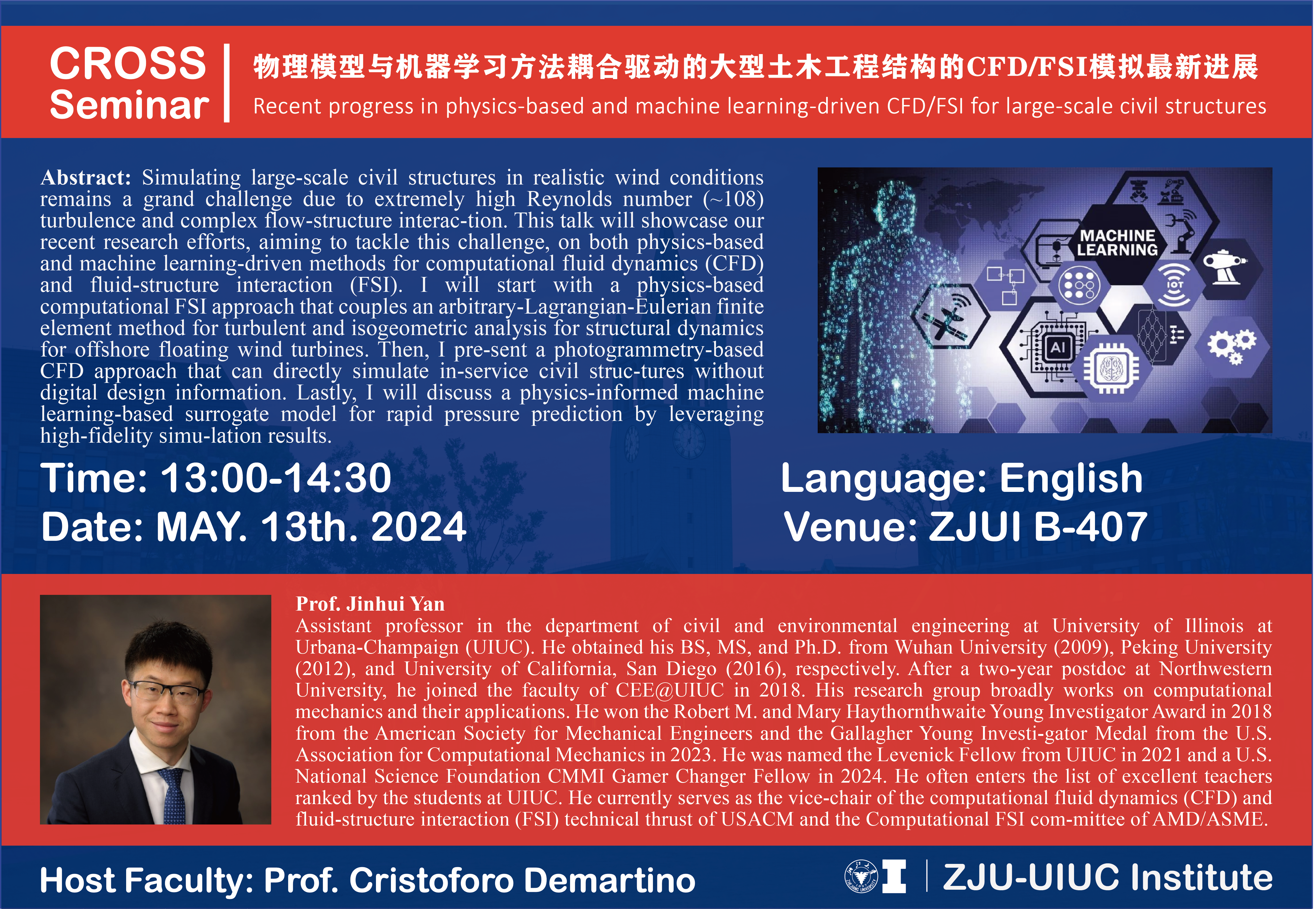
Bio: Jinhui Yan is an assistant professor in the department of civil and environmental engineer-ing at University of Illinois at Urbana-Champaign (UIUC). He obtained his BS, MS, and Ph.D. from Wuhan University (2009), Peking University(2012), and University of California, San Diego (2016), respectively. After a twoyear postdoc at Northwestern University, he joined the faculty of CEE@UIUC in 2018.His research group broadly works on computational mechanics and their applications. He won the Robert M. and Mary Haythornthwaite Young Investigator Award in 2018 from the American Society for Mechanical Engineers and the Gallagher Young Investigator Medal from the U.S. Association for Computational Mechanics in 2023. He was named the Levenick Fellow from UIUC in 2021 and a U.S. National Science Foundation CMMI Gamer Changer Fellow in 2024. He often enters the list of excellent teachers ranked by the stu-dents at UIUC. He currently serves as the vice-chair of the computational fluid dynamics (CFD) and fluid-structure interaction (FSI) technical thrust of USACM and the Computational FSI committee of AMD/ASME.
Abstract:
Simulating large-scale civil structures in realistic wind conditions remains a grand challenge due to extremely high Reynolds number (~108) turbulence and complex flowstructure interac-tion. This talk will showcase our recent research efforts, aiming to tackle this challenge, on both physics-based and machine learning-driven methods for computational fluid dynamics (CFD) and fluid-structure interaction (FSI). I will start with a physics-based computational FSI approach that couples an arbitrary- Lagrangian-Eulerian finite element method for turbulent and isogeometric analysis for structural dynamics for offshore floating wind turbines. Then, I pre-sent a photogrammetry-based CFD approach that can directly simulate in-service civil structures without digital design information. Lastly, I will discuss a physics-informed machine learning-based surrogate model for rapid pressure prediction by leveraging high-fidelity simu-lation results.